Confirmed Speakers
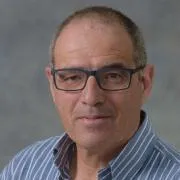
Meir Feder (ITSoc Padovani Lecturer)
Title: Addressing Large Models: Multiple and Hierarchical Universality
Abstract: Universal coding, prediction and learning usually consider the case where the data generating mechanism is unknown or non-existent, and the goal of the universal scheme is to compete with the best hypothesis from a given hypothesis class, either on the average or in the worst-case. Multiple universality considers the case where the relevant hypothesis class is also unknown: there is a set of hypotheses classes, possibly with different complexities. Sometime, but not necessarily, simpler classes are nested within more complex classes. A main challenge is to correctly define the universality criterion so that the extra “regret” for not knowing the relevant class is monitored. We propose several possible definitions and derive their min-max optimal solutions, including the suggestion of an hierarchy of such sets. Interestingly, the proposed approach can be used to obtain Elias codes for universal representation of the integers. Further, we suggest a multiple universality approach for general linear models, including linear regression, logistic regression and Perceptron. Finally, we present how multiple universality, with its non-uniform convergence and regret bounds, can be applied to explain and design learning schemes for general, large or even huge, “over-parameterized” model classes such as deep neural networks, transformers and so on.
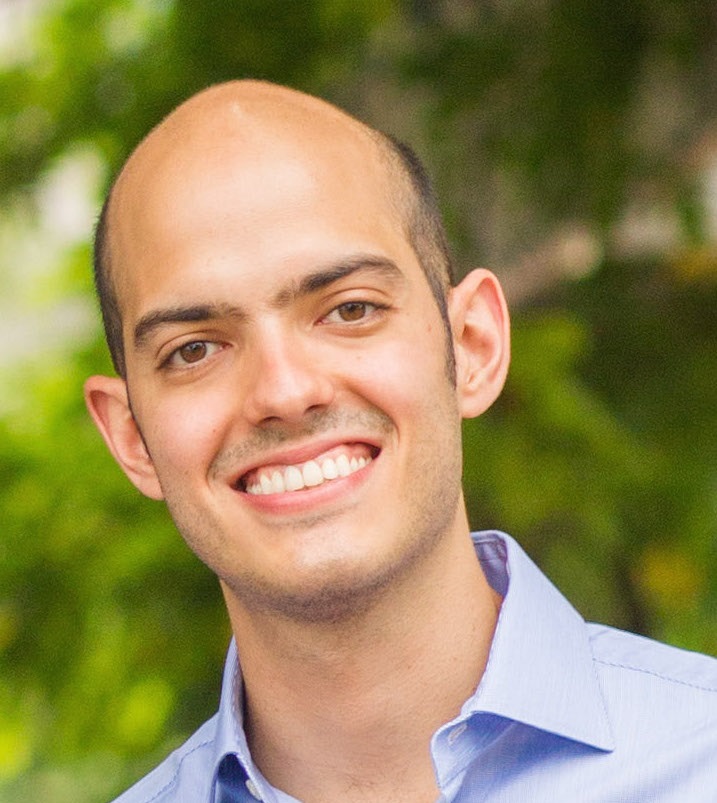
Flavio Calmon
Title: A World of Divergences
Abstract: This talk presents information-theoretic results on three facets of trustworthy machine learning: fairness, arbitrariness, and privacy. We first broadly discuss the landscape of fairness interventions in classification and prediction tasks and present a post-processing technique, “FairProjection,” designed to ensure group fairness in prediction and classification. We also discuss converse results based on Blackwell’s “comparison of experiments” theorem that captures the limits of group fairness assurance in classification. These results show that existing techniques (including FairProjection) can approach the optimal Pareto frontier between accuracy and group fairness in specific settings. We then review the concept of predictive multiplicity in machine learning. Predictive multiplicity arises when different classifiers achieve similar average performance for a specific learning task yet produce conflicting predictions for individual samples. We present recent metrics and findings on multiplicity and their implications for applications such as content moderation. We then briefly highlight recent findings at the interface of information theory and differential privacy, including new methods for privacy accounting and the design of privacy mechanisms. We conclude with future research directions in responsible machine learning and artificial intelligence.
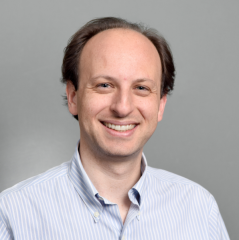
Oliver Kosut
Title: An Information-theoretic Perspective on Privacy Measures
Abstract: How do you measure information? This question has been central to classical information theory problems, such as data compression and channel coding, and it is also crucial to the modern problem of data privacy. If sensitive data is used to train a learning model, or perform inference, how much private information has been revealed? Privacy differs dramatically from secrecy in that perfect privacy (i.e., close to zero mutual information) is impossible, because some information must be taken from the data. Instead, we must choose a privacy measure, and find how much information has been revealed according to that measure. The first half of the talk will cover a broad selection of information measures, including f-divergences and Rényi divergences, both of which are generalizations of the Kullback-Leibler divergence. The second half will focus on privacy, and how most privacy measures can be viewed as special cases of these information measures. I will discuss the many variants of differential privacy, as well as maximal leakage and maximal alpha-leakage. Finally, I will conclude with a discussion of optimal privacy mechanisms, and recent work deriving optimal mechanisms for differential privacy based on an information-theoretic framework analogous to classical rate-distortion.
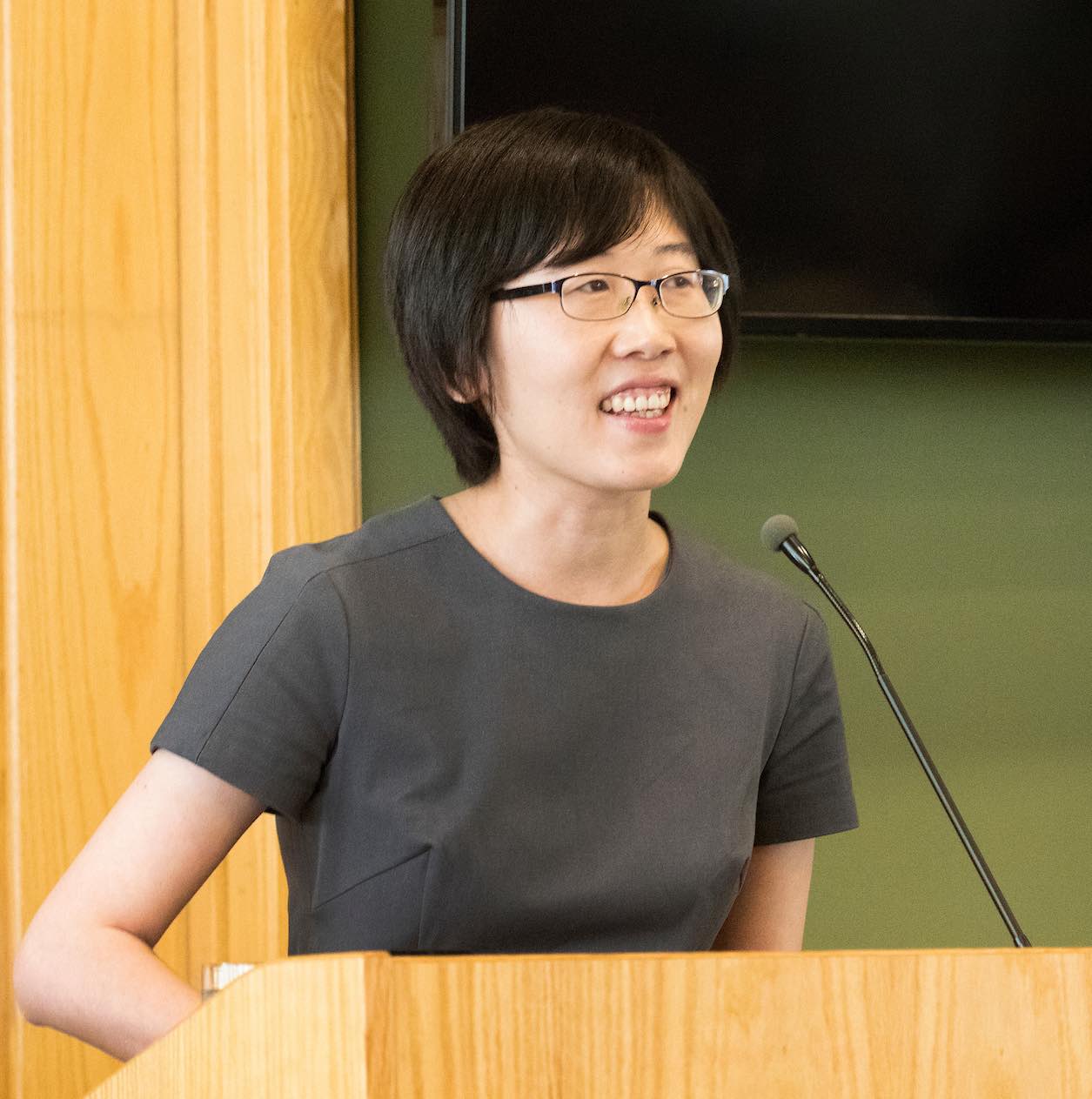
Yuejie Chi
Title: Generative Priors in Data Science: From Low-rank to Diffusion Models
Abstract:Generative priors are effective countermeasures to combat the curse of dimensionality, and enable efficient learning and inversion that otherwise are ill-posed, in data science. Focusing on the statistical and algorithmic underpinnings, the tutorial discuss two ubiquitous types of priors for solving inverse problems: low-rank models, which postulate the signal of interest lie in some low-dimensional subspace, and score-based diffusion models, which prescribe the score functions of the marginal distributions of a noise-diffused forward process.
Through the lens of matrix and tensor factorization, the tutorial illuminates the optimization geometry of nonconvex low-rank factorization with the aid of statistical reasoning, and how gradient descent harnesses such geometry in an implicit manner to achieve both computational and statistical efficiency all at once. To further combat with ill-conditioning, we introduce scaled gradient descent (ScaledGD), a method that provably converges linearly at a constant rate independent of the condition number at near-optimal sample complexities, while maintaining the low per-iteration cost of gradient descent, even when the rank is overspecified and the initialization is random.
Going beyond low rank, the tutorial develops a suite of non-asymptotic theory towards understanding the data generation process of diffusion models in discrete time for both deterministic and stochastic samplers, assuming access to inaccurate estimates of the score functions. We further discuss how to provably accelerate data generation without additional training, leveraging higher-order approximation. Last but not least, we introduce a plug-and-play method that is provably robust for nonlinear inverse problems using unconditional diffusion priors.
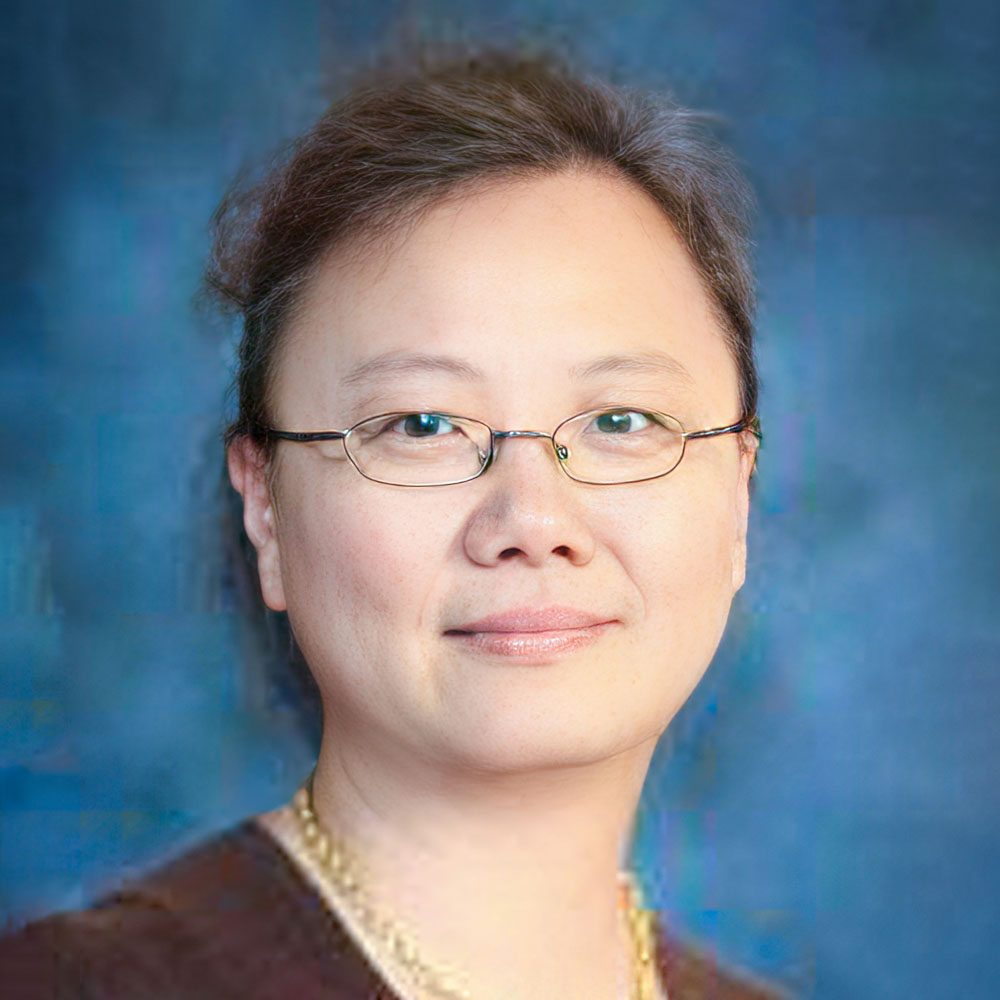
Yingbin Liang
Title: Theory on Transformer Training
Abstract: Transformers, as foundation models, have recently revolutionized many machine learning (ML) applications such as natural language processing, computer vision, robotics, etc. Alongside their tremendous experimental successes, theoretical studies have emerged to explain why transformers can be trained to achieve the desired performance. This tutorial aims to provide an overview of these recent theoretical investigations that have characterized the training dynamics of transformer-based ML models. Additionally, the tutorial will explain the primary techniques and tools employed for such analyses. Specifically, the tutorial will begin with an introduction to basic transformer models, and then delve into several ML problems where transformers have found extensive application, such as in-context learning, next token prediction, and unsupervised learning. For each learning problem, the tutorial will go over the characterization of the training process, the convergence guarantee, and the optimality and insights of the attention solution. Finally, the tutorial will discuss future directions and open problems in this actively evolving field.
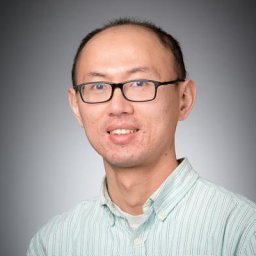
Jun Chen
Title: Lossy Data Compression using Deep Learning
Abstract: Deep generative models when applied to (lossy) image compression tasks can reconstruct realistic looking outputs even at very low bit-rates, when traditional compression methods suffer from significant artifacts. This has led to a significant interest in both the information theoretic aspects, as well as practical architectures of deep learning based image compression.
In this tutorial we will provide a survey of existing deep learning based compression systems that have appeared in the literature over the past few years. We will introduce the concept of perception loss that appears naturally when training deep learning based models. We will then introduce the rate-distortion-perception (RDP) function that underlies the performance of learned image compression systems and explain its connection to the classical rate-distortion function in information theory. We will discuss several properties of the RDP function and study the quadratic Gaussian source model in detail. Motivated by the Gaussian case, we will introduce a notion of universal encoded representations, where the same compressed representation can be used to simultaneously achieve different operating points on the distortion-perception trade-off. We will demonstrate through both theoretical analysis and experimental results involving deep learning models that near-optimal encoding representations can be constructed for a broad class of source models.
Building upon the insights from RDP function, we will consider a closely related setup — cross-domain lossy compression — that applies to a broader class of applications involving image restoration tasks (e.g., de-nosing, super-resolution etc.) over compressed data representations. We will establish coding theorems that rely on recent one-shot methods in information theory, establish connections to the problem of optimal transport, and develop architectural insights that are validated using deep learning models.
Different from conventional lossy source coding for which it suffices to consider deterministic algorithms, cross-domain lossy compression generally has to resort to stochastic mappings and can also benefit from the availability of common randomness. We will elucidate the role of randomness and how it depends on the formulation of perception constraint.
Finally, we will consider an information-theoretic objective, termed information constrained optimal transport, that arises from cross-domain lossy compression. Its functional properties, asymptotics and bounds will be discussed. The quadratic vector Gaussian case will be leveraged as an illustrative example to show that this line of research has the potential to generate many theoretical results with practical implications.
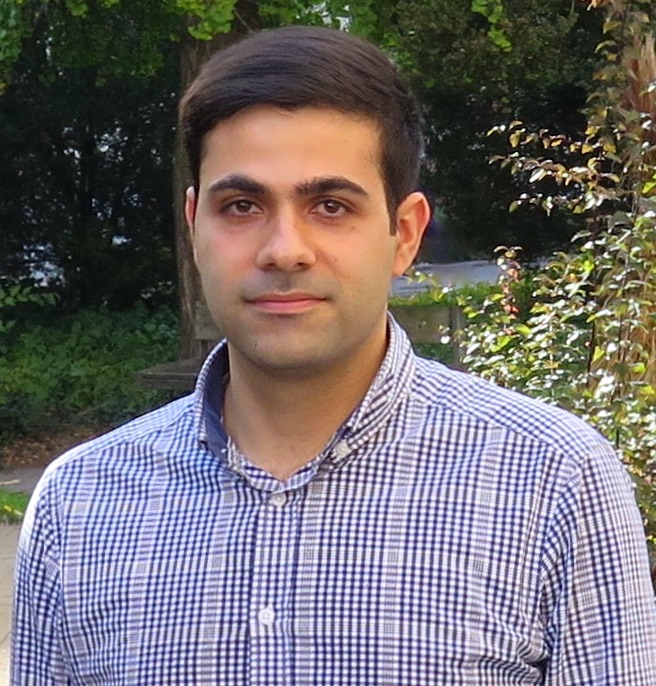
Hamed Hassani
Title: Alignment of Machine Learning Models: From CNNs to LLMs
Abstract: My goal in this talk is to give an overview of how the machine learning community has thought about the trustworthiness of trained models. This topic has been studied from several perspectives including the adversarial setting which is the focus of this talk. I'll start with adversarial examples and then make a (natural) transition to the recently emerged area of AI alignment wherein the broad objective is to “align” the output text generated by LLMs with ethical and legal standards. I will cover my research in these areas during the last few years ranging from attacks and defenses to fundamental limits and new notions of robustness. The talk will be self-contained and no particular background on the alignment of machine learning models will be needed.
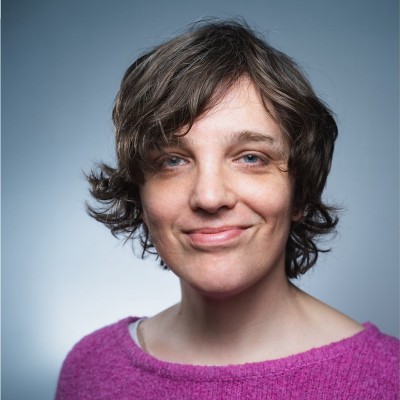
Jona Ballé
Title: Learned Data Compression, and New Solutions to Old Problems
Abstract: Since its emergence roughly 7 years ago, the field of learned data compression has attracted considerable attention from both the machine learning and information theory communities. Data-driven source coding promises faster innovation cycles, as well as better adaptation to novel types of sources and unconventional models of distortion. For example, image codecs can now be end-to-end optimized to perform best for specific types of images, by simply replacing the training set. They may now be designed to minimize a given perceptual image metric, or in fact any differentiable perceptual loss function. In this talk, I will review nonlinear transform coding (NTC), a framework of techniques which over the past few years have superseded the state of the art of hand-crafted image compression methods (such as the family of JPEG and MPEG standards) in terms of subjective quality vs. rate. I'll illustrate the empirical rate-distortion performance of NTC with the help of simple, analytically characterized data sources. Furthermore, I will discuss two directions of ongoing work, both of which use machine learning to address long-standing, hard research problems: First, better measures of perceptual quality, as captured by realism ("How realistic is an image?") and fidelity ("How similar is an image to a reference?"). We discuss Wasserstein Distortion, a measure to unify the two, grounded in neuroscientific models of peripheral vision. Second, practical schemes for distributed lossy compression; in particular, the Wyner-Ziv problem, in which the decoder has direct access to correlated information that is unknown at the encoder. We show that for some well-studied source distributions, learned compressors mimic optimal solutions such as "binning" or "grouping" in the source space. These interpretable behaviors appear even though no particular structure is imposed, and - as usual for data-driven methods - no knowledge of the source distribution is assumed, only the availability of samples.
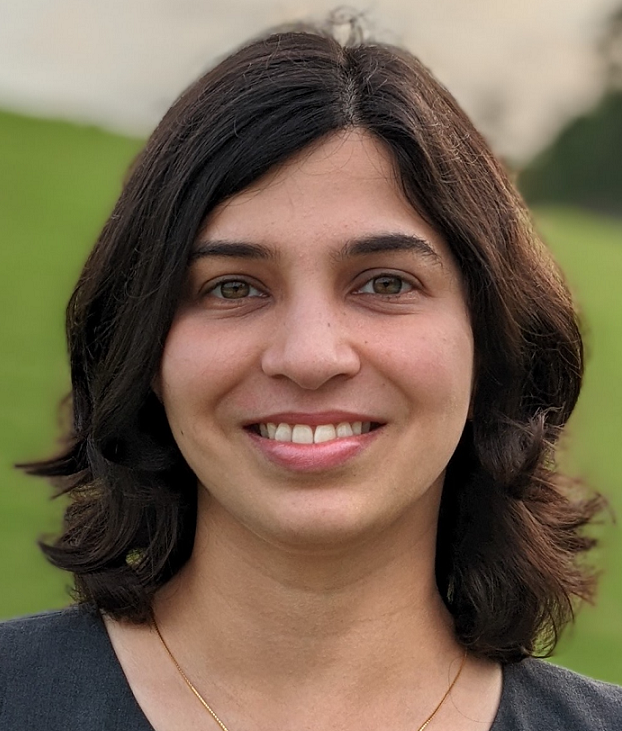
Gauri Joshi
Title: Federated Learning in the Presence of Data, Communication and Computational Heterogeneity
Abstract: A key aspect that sets federated learning apart from data-center-based distributed training is the inherent data, communication, and computation heterogeneity across the edge clients. Allowing heterogeneity is essential for the system to be scalable and flexible. However, heterogeneity can cause convergence slowdown and inconsistency problems for federated optimization algorithms. In this tutorial, you will learn how to design and analyze optimization algorithms for tackling various types of heterogeneity in federated learning. We will also discuss some open problems and connections to information theory.
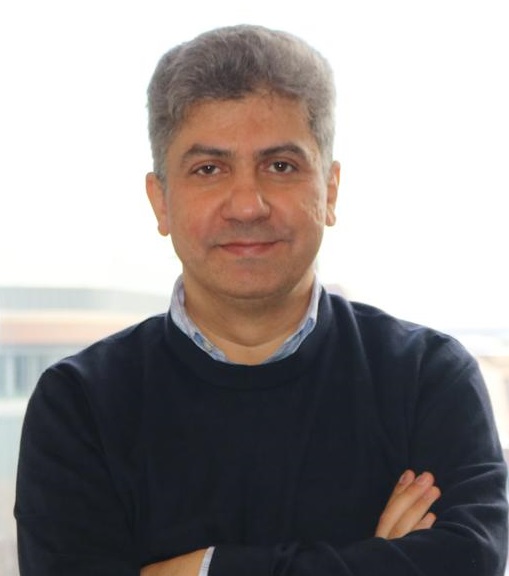
Mohammad Ali Maddah-Ali
University of Minnesota Twin Cities
Title: Blockchains, Decentralized Learning, and Game of Coding
Abstract: The decentralization movement, which emerged in 2008 with the success of Bitcoin, aims to revolutionize digital platforms by making them transparent and open to the public for coordination, contribution, and verification. This movement is currently reshaping the AI landscape, with a focus on designing decentralized machine learning (DeML) platforms. However, blockchains, as decentralized trust engines, face limitations in computational power, which hinder this transformation.
In this talk, we will first review blockchain consensus, its potential to enable distributed computing platforms, and its inherent limitations. We will then discuss why existing solutions based on verifiable computation outsourcing are ineffective for massive and approximate ML computations. As an alternative, we will introduce the game of coding, which leverages the power of redundant (coded) computing to effectively detect and correct errors in outsourced tasks. The game of coding utilizes the inherent rationality of potential adversaries to expand the applicability of coding theory in a trust-minimized setting, where external nodes are overwhelmingly dishonest.
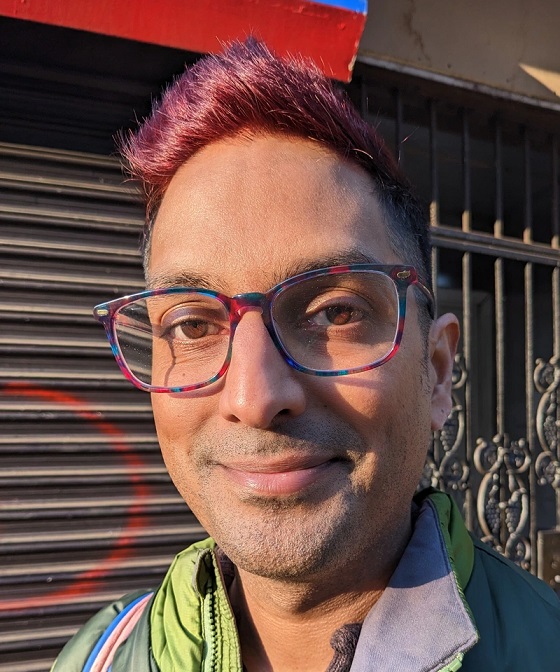
Anand Sarwate
Title: Learning with Structured Tensor Decompositions
Abstract: Many measurements or signals are multidimensional, or tensor-valued. Vectorizing tensor data for statistical and machine learning tasks often results in having to fit a very large number of parameters. Using tensor decompositions to model such data can give a flexible and useful modeling framework whose complexity can adapt to the amount of data available. This talk will introduce classical decompositions (CP, Tucker) as well as more recent ones (tensor train, block tensor decomposition, and low separation rank) and show how they can be used to learn scalable representations for tensor-valued data and make predictions from tensor-valued data. Along the way, we will highlight some applications as well as open problems for future research.